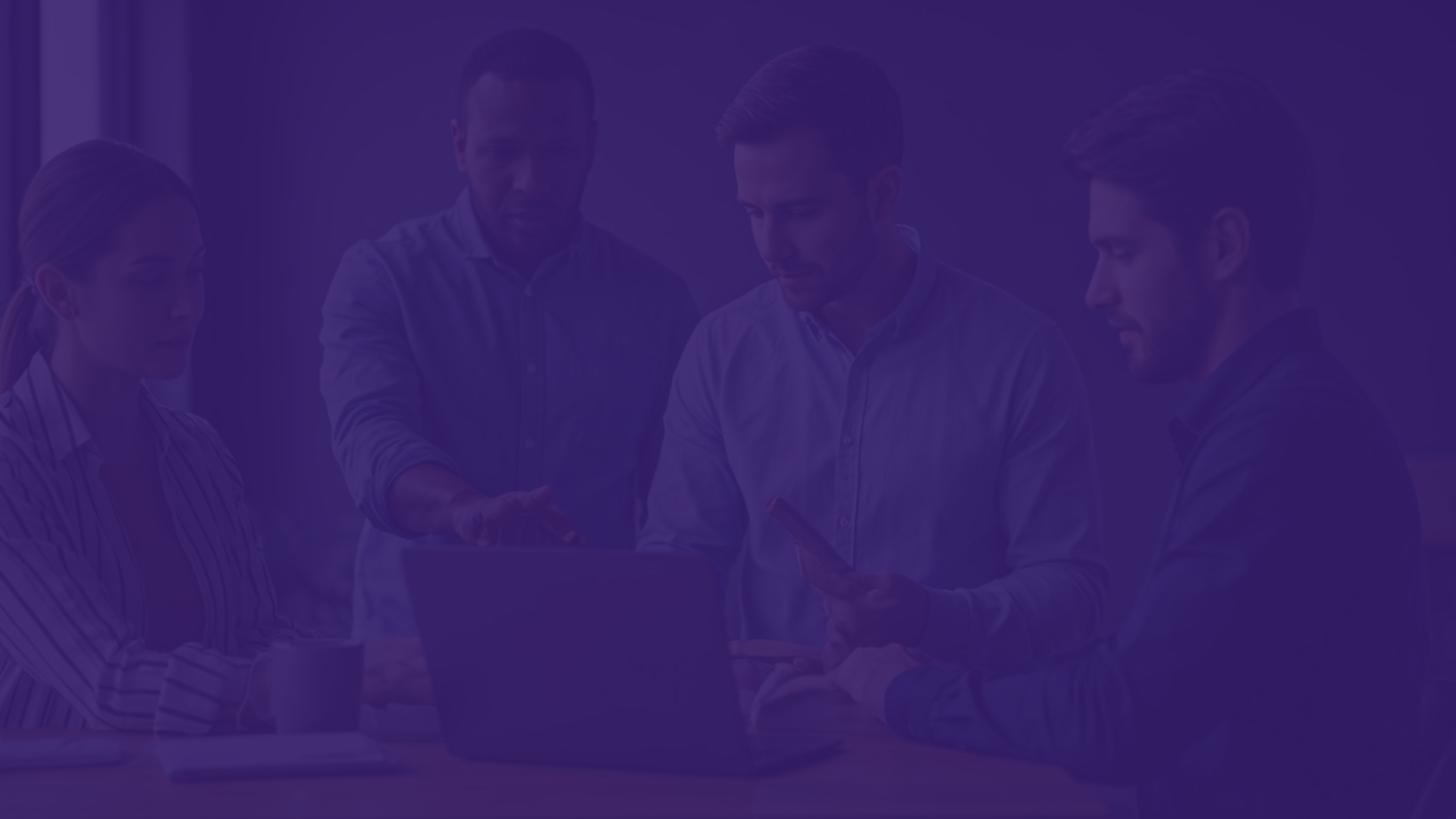
How AI is Transforming Data Analytics: A Deep Dive into Big Data
In today’s digital age, data visualization, and data are essential for businesses. Data analytics is now a key part of how organizations work, no matter their field. There is a huge amount of data created every day, and this has changed the way data analytics is done. Artificial intelligence (AI) is now helping to transform this area. This integration of AI and data analytics is changing how businesses collect, analyze, and understand information. This change helps them make smarter choices and reach new heights in efficiency and creative work.
ARTIFICIAL INTELLIGENCE (AI)
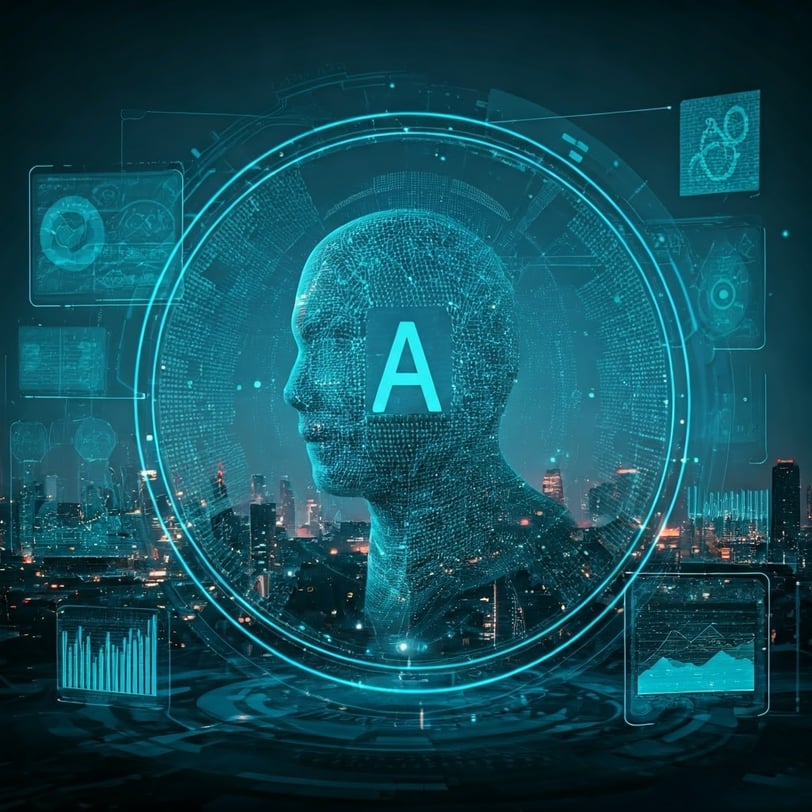
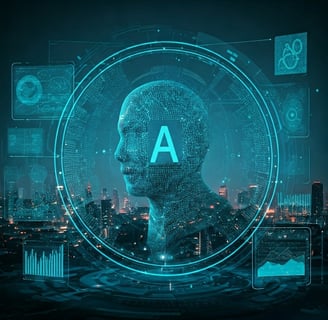
Key Highlights
AI is revolutionizing data analytics, enabling businesses to gain deeper insights from their data than ever before.
Machine learning algorithms, deep learning, natural language processing, and other AI-powered technologies are automating data analysis tasks, making them faster, more accurate, and more scalable.
AI is being used to improve customer experiences, optimize supply chains, enhance healthcare outcomes, and detect financial fraud.
As AI technology continues to evolve, the future of data analytics looks bright.
By understanding the key trends and challenges of AI in data analytics, businesses can position themselves to fully leverage the power of this transformative technology.
Introduction
In today’s digital age, data visualization, and data are essential for businesses. Data analytics is now a key part of how organizations work, no matter their field. There is a huge amount of data created every day, and this has changed the way data analytics is done. Artificial intelligence (AI) is now helping to transform this area. This integration of AI and data analytics is changing how businesses collect, analyze, and understand information. This change helps them make smarter choices and reach new heights in efficiency and creative work.
Exploring How AI Revolutionizes Data Analytics and Big Data
Traditionally, data analysis used to rely a lot on manual work and statistical methods. This made it slow and limited how much information could be gathered quickly. But now, with the rapid growth of AI technologies, especially in machine learning and deep learning, we are experiencing a big change in data analytics and its various use cases. AI can handle vast amounts of data, find complicated patterns, and create actionable insights. This has greatly changed how businesses work with data.
AI is helping businesses predict customer behavior and improve supply chains. It is changing many parts of data analytics. By taking care of boring and repetitive tasks, AI allows data experts to concentrate on more important goals, like finding new business opportunities and leading data-driven innovation.
1. The Role of AI in Enhancing Data Collection Techniques
Data collection is the first step in data analytics and effective data management, and AI has made this process much better. In the past, businesses only used structured data from databases and spreadsheets. Now, there is a lot of unstructured data available, like social media posts, customer reviews, and emails. AI systems, especially those that use natural language processing, can analyze and pull valuable insights from these sources.
AI tools can gather data from different places without human help. This saves time and money while giving a full view of the data. For example, AI web scraping tools can pull data from websites, and social media monitoring tools can track brand mentions and feelings about products.
This improved data collection is very important today. Businesses must understand customer feelings and market trends quickly. With AI, companies can see all of their data clearly, which helps them make smart decisions and stay ahead of others.
2. Advanced Data Processing: AI's Contribution to Speed and Efficiency
Once we collect data, we need to process it before we can analyze it. This step is called data preprocessing. It involves cleaning the data and changing it into a format we can use. We also need to fix any missing values. AI plays a big role in making data processing faster and easier.
AI models can take care of many tasks in data preprocessing. They can clean the data, change it, and create new features. These models can find and fix missing values, get rid of duplicate entries, and turn data into the right format for analysis. This helps reduce the time and work needed for data preparation.
With this better efficiency in data processing, data analysts can focus on more complex tasks. They can build models and interpret the data. By using AI and AI’s ability to handle the harder parts of data analysis, organizations can understand their data more quickly. This helps them react to changes in the market and make smart, data-driven choices faster.
3. AI-Driven Data Analysis Methods: From Predictive Analytics to Deep Learning
AI has changed how we analyze data. It provides strong tools like predictive analytics and deep learning. These tools help find insights that were once hidden in large amounts of data points. Predictive analytics, which uses AI algorithms, helps businesses guess future trends. This is done by looking at past information. Businesses can forecast things like customer departures or stock market changes. AI predictive models are getting better at detecting hidden patterns and offering useful predictions.
Deep learning is another key player in data analysis. It is part of machine learning and takes inspiration from how the human brain works. Deep learning algorithms are very good at spotting complex patterns and connections in data. This skill has led to big advances in areas such as image recognition and natural language processing. It allows businesses to get insights from tricky data sources like images, written text, and voice recordings.
By using the combined strength of predictive analytics and deep learning, businesses can dig deeper into their data. They can find detailed relationships and gain insights that help with smart decision-making and spark innovation.
4. The Impact of Machine Learning on Data Interpretation and Decision Making
Interpreting data analysis results and turning them into useful insights is very important for good decision-making. Machine learning is changing how we look at data and is making it easier for more people in organizations to understand. Machine learning models can spot important trends, unusual results, and patterns in data. They show which areas need more attention.
These models can also create visuals and dashboards that make complex data easy to follow. This helps users who aren't technical understand what the analysis means. Plus, machine learning can automate making reports and summaries. This saves analysts a lot of time and effort.
By making data easier to understand, machine learning helps businesses make smarter decisions based on data. It stops organizations from relying only on gut feelings and stories. Instead, they can use insights backed by data to guide their plans and actions.
5. Integrating AI with Big Data for Real-time Analytics and Insights
In today's world of big data, analyzing large amounts of data quickly is very important for making good decisions. AI helps businesses by enabling them to analyze data in real-time and providing useful insights as events happen. AI platforms can look through and understand huge datasets from many sources, like social media feeds, sensor data, and transactions, much faster than before.
This ability helps businesses keep an eye on key metrics in real-time, spot new trends, and find problems as they come up. For example, e-commerce companies can use real-time analytics to tailor customer experiences, change prices on the fly, and manage inventory based on current demand.
With AI-driven real-time analytics, businesses are changing how they work. They can shift from waiting for issues to arise to making decisions beforehand. By using AI and big data effectively, companies can gain an edge over their competition by making choices based on data that fits the constantly changing market.
6. Navigating the Challenges: AI in Addressing Data Privacy and Security
As businesses use AI more for data analytics, it is very important to deal with data privacy and security issues. AI algorithms often need sensitive information, which raises worries about data breaches and unauthorized access. The good news is that AI can also help improve data privacy and security. It can provide new ways to reduce risks and protect important information.
AI algorithms can be trained to find potential data breaches and notice unusual activities. They can make security protocols stronger too. AI is essential for keeping personal data private and making sure companies follow rules like GDPR and CCPA. For example, AI tools can find and remove sensitive details from documents, encrypt data, and keep an eye on access logs for any strange activities.
By using AI for threat detection, anomaly detection, and data protection, businesses can better handle the challenges of data privacy and security. This helps companies create stronger security systems, keep sensitive information safe, and maintain customer trust in a world that relies more on data.
Key Technologies Behind AI in Data Analytics
AI is changing the way we use data analytics. Several important technologies make this change possible. They help businesses find useful insights in vast amounts of data. This allows them to make better decisions.
One of these key technologies is machine learning. It lets computers learn from data without needing detailed programming. With machine learning, computers can spot patterns, predict outcomes, and automate tasks. This is changing how businesses look at and understand data.
1. Machine Learning Algorithms and Their Applications
Machine learning algorithms are crucial for AI's impact on data analytics. These algorithms help computers learn from data. They can find patterns and make predictions without being programmed for each task. They are used in many areas, like predicting customer loss or spotting fraud.
One key use of machine learning in data analytics is predictive modeling. These algorithms look at past data to see patterns and links. This helps to guess what might happen next, like how customers will behave or if machines will fail. This ability to predict helps businesses make smart choices, reduce risks, and improve their work.
Machine learning algorithms also help with tasks like clustering, classification, and anomaly detection. This gives businesses tools to group customers, sort data, and find unusual cases. These skills are very helpful in fields such as marketing, finance, healthcare, and manufacturing. They guide businesses to make choices based on data, which fosters growth and innovation.
2. Natural Language Processing for Enhanced Data Understanding
Natural language processing (NLP) is a part of AI. It helps computers understand, interpret, and create human language. NLP is changing data analytics by allowing us to derive insights from the huge amounts of textual data we create every day. This textual data comes from social media posts, customer reviews, news articles, and emails.
Sentiment analysis is an important use of NLP. It helps businesses understand public opinion and customer feelings about their brand, products, and services. By looking at texts for positive, negative, or neutral feelings, businesses can learn a lot about customer satisfaction. They can also spot possible PR issues and make smart decisions to improve their products and services.
Here are some other ways NLP helps with understanding data:
Text Summarization: Creating short summaries of long documents. This helps people process information faster and make better decisions.
Chatbots: Managing customer service chats automatically. This gives support all day, every day, and allows human workers to handle more complicated issues.
Information Extraction: Pulling out important names, links, and facts from messy text. This helps in discovering new knowledge and improving data.
3. Neural Networks: Powering Complex Data Analysis Tasks
Neural networks are inspired by how the human brain works. They are a strong type of machine learning algorithm. These networks are made up of connected nodes that are arranged in layers. This structure lets them handle a lot of information and learn complex patterns.
Neural networks perform very well in tasks like image recognition, natural language processing, and speech recognition. They do better than traditional methods in terms of accuracy and efficiency. In data analytics, neural networks are useful for many things, like fraud detection, risk assessment, and giving personalized recommendations. Their skill in processing complex data makes them great for analyzing high-dimensional data and finding subtle connections.
As neural networks grow and improve, they will become even better at managing complex data analysis tasks. This progress will create new opportunities for businesses. They will be able to gain deeper insights from their data and streamline complicated decision-making processes.
The Significance of AI in Various Stages of Data Analytics
The impact of AI is clear in every part of data analytics. It starts with data cleaning and goes all the way to creating actionable insights and making strategic decisions.
AI can automate boring and repetitive tasks. This includes data cleaning and normalization. Because of this, data experts can spend time on more complex tasks that add more value.
1. Data Preprocessing and Cleansing with AI Tools
Data preprocessing and cleaning are important parts of the data analytics process. The insights we get from data rely heavily on the quality of that data. AI tools are helpful for automating and improving data preprocessing and cleaning. They make sure the data is accurate, consistent, and ready to be analyzed.
AI-powered data cleaning tools can quickly find and fix missing values. They can also detect and correct errors, remove duplicates, and make data formats uniform. These tools can spot and manage outliers, which helps keep analysis results accurate.
By automating these jobs, AI tools save time for data professionals. They also reduce the chances of human errors, leading to better and more reliable results. This lets data professionals spend more time on important tasks like feature engineering and model selection. As a result, decision-making can become better.
2. AI in Data Mining: Discovering Patterns and Insights
Data mining is about finding useful information and patterns in large sets of data. AI makes this process much better. AI algorithms can check huge amounts of data, discover hidden patterns, and find connections that are hard for people to see.
Machine learning algorithms are great for data mining. They can do tasks like grouping data, sorting data into specific types, and finding patterns showing how different factors relate to each other. This helps businesses gain valuable insights about their customers, processes, and market trends.
With AI automating the search for patterns, businesses can make better choices, improve their processes, and get ahead of their competition. AI helps them move from simply looking back at past events to predicting future ones and suggesting the best actions to take.
3. Predictive Analytics: Forecasting Future Trends with AI
Predictive analytics and prescriptive analytics are strong parts of AI. Predictive analytics helps businesses predict future trends very accurately. By using machine learning and predictive models, companies can find valuable insights from large datasets. AI algorithms look for patterns in data and can guess outcomes that help business success. The integration of AI in predictive analytics is a big change from traditional methods. It gives better predictions and deeper insights into various industries. With AI technology leading the way, forecasting has changed. Now, businesses can wisely deal with changing market conditions.
Real-world Applications of AI in Data Analytics
AI is changing the game in data analytics. It is not just something that will happen in the future. It is here and now, impacting many industries. AI helps improve customer experiences and makes supply chains work better. It is solving real problems, making businesses do well, and bringing new ideas to different fields.
A key area where AI helps is in customer relationship management (CRM). AI tools can look at customer data. They give personalized suggestions, automate marketing tasks, and make customer service interactions better.
1. Enhancing Customer Experiences through AI-Powered Analytics
In today's tough market, giving great customer experiences is very important for success. AI-powered tools are changing how businesses manage customer experiences. They help companies make personal connections, predict what customers want, and build strong relationships. By using AI algorithms, businesses can look through vast amounts of customer data. This includes things like what people buy, how they browse, and their feedback. This helps businesses understand what their customers like and need.
For example, AI recommendation tools are very common in online shopping. They suggest products based on what a customer bought before and what they looked at. This kind of personalization makes customers happier and also boosts sales and loyalty.
AI can also check customer feedback from different places, like social media and surveys. It helps find issues and fix them before they become bigger problems. By using this proactive method, businesses can focus on what customers really want. This leads to better customer satisfaction, loyalty, and customers who are willing to share their positive experiences.
2. AI in Healthcare: Improving Patient Outcomes with Data Insights
AI in healthcare has changed how patients do it. It uses data to help doctors and hospitals make better choices across various sectors. With machine learning and predictive analytics, healthcare workers can spot diseases early. They can also create treatment plans that fit each patient. AI systems look at vast amounts of medical data. This helps in making better and quicker decisions. By using AI technologies, hospitals can run smoothly, make fewer mistakes, and work better overall. AI also helps in predictive modeling and risk management. It has a big effect on healthcare, leading us into a time where care is based on data and focused on patients.
3. AI-Enhanced Financial Services: Risk Assessment and Fraud Detection
In the world of financial services, AI is changing how we assess risk and find fraudulent activities and fraud. Using machine learning and data analytics, AI systems can spot unusual patterns and detect fraud in large datasets. This new technology gives valuable insights to help reduce risks and improve security. With predictive models and advanced algorithms, AI helps organizations manage risks and protect against financial fraud. The integration of AI in financial services marks a big change. It provides accurate predictions and supports organizations in making strategic decisions thanks to its innovative features.
4. Optimizing Supply Chains: AI’s Role in Logistics and Inventory Management
AI is changing how supply chains work. It helps make things run better, costs lower, and customers happier. AI looks at data from many sources like sensors, GPS trackers, and weather reports. This way, supply chain systems powered by AI can find problems, predict issues, and improve routes. Because of this, deliveries are on time, transport costs go down, and surprises have less impact.
In managing inventory, AI algorithms check old sales data and trends. They also look at outside factors to guess what will be needed in the future and keep stock levels right. This means businesses have just enough inventory to handle what customers want without spending too much on storage or running out of products.
By using AI to study complex data and find patterns, companies can streamline their supply chain. This includes everything from sourcing items to delivery, making the whole process more reliable, quick, and profitable.
The Future of Data Analytics with AI
The fast changes in AI are greatly affecting the future of data analytics, introducing a paradigm shift. Companies that do not keep up with these changes may fall behind and lose important chances. As AI keeps getting better, we should see more advanced ways to analyze data. This will help businesses understand things better and make intelligent choices.
In the future, data analytics will have more automation. There will be easier analytics tools for users who are not tech experts. There will also be more attention on ethical issues like data privacy and bias in algorithms.
1. Emerging Trends in AI and Big Data Integration
The combination of AI and big data is an important new trend in data analytics. It helps businesses to use large amounts of data from various sources for better insights and decisions. AI algorithms are key for looking at and understanding the huge amounts of data that we have today. This leads to smarter business intelligence and choices based on data.
As data gets more complex and plentiful, companies are choosing cloud-based AI systems to improve their analytics. They want to take advantage of the newest AI technology. This trend towards using cloud AI is predicted to grow. It will give businesses more flexibility, scalability, and savings in their data analytics.
Also, edge computing is coming up. This is when data is processed closer to where it comes from. It offers new chances for real-time analytics and choices. AI will be very important in this setup by allowing smart devices at the edge to process data locally and make their own decisions.
2. The Role of Ethics in AI-Driven Data Analytics
As AI becomes more important in data analytics, it is very vital to think about ethics. Businesses need to develop and use their AI systems in a responsible and honest way. This helps to build trust with customers and other people involved.
One main ethical issue with AI in data analytics is bias. AI algorithms only work well if they have good training data. If the data is biased, the AI models can also be biased. This can cause unfair results in important areas like hiring, lending, and criminal justice.
To reduce bias, businesses must focus on having a mix of data, fairness, and accountability in their AI systems. This means they should pick training data carefully to lessen bias. They should also use fairness metrics to check their AI models for discrimination. Lastly, it is important to provide clear reasons for how AI systems come to their decisions.
3. Future Skills: Preparing the Workforce for an AI-Dominated World
As AI changes the world of data analytics, we must get the workforce ready with the right skills and knowledge. Future workers will need a mix of technical skills and people skills. This combination will help them make the most of AI and handle the ethical issues that come with more automation.
Jobs for data professionals, like data analysts, data scientists, and machine learning engineers, will stay in high demand. However, their jobs will change. They will need to understand AI algorithms, data engineering basics, and ethical concerns better. Training programs to improve current workers' skills will be important to meet the new needs in data analytics.
Also, it will be crucial to build skills like critical thinking, creativity, communication, and teamwork in an AI-focused world. While AI can automate tasks and analyze data, human qualities like intuition, empathy, and judgment are still vital. These traits help understand insights, make ethical choices, and encourage innovation.
Conclusion
In the world of data analytics, AI is changing everything. It helps us collect, process, and analyze large amounts of data. With smart machine learning methods and neural networks, AI makes work easier and provides quick insights. This is helpful for different industries, like healthcare, finance, and supply chain management.
As we look to the future with AI, we must think about ethics and preparing the workforce. It is important to connect AI abilities with human understanding. This will help us get the most out of data analytics. By tackling technical challenges and being clear in our actions, AI can greatly help businesses become more efficient and spark new ideas. As we go through this AI-driven world, combining what humans know with AI technology will lead to amazing breakthroughs in data analytics.
Frequently Asked Questions
How is AI transforming traditional data analytics processes?
AI is changing the way we look at data analytics. It automates old methods, which makes things more accurate. This helps businesses handle large amounts of data better, revealing deeper insights. As a result, they can get faster and more precise outcomes. This allows for a better understanding of complex data.
What are the ethical considerations in using AI for data analytics?
Ethical issues in AI data analytics include caring for data privacy. It also means protecting against biased algorithms that can wrongly interpret consumer behavior. Additionally, it is important to be clear about how data is collected and used.
Can small businesses leverage AI in data analytics effectively?
Yes, many affordable AI data analytics tools are out there. They help small businesses get useful insights from their data. This can give them an edge over the competition.
What are the potential risks of relying heavily on AI for data analytics?
AI improves data analytics. However, depending too much on it without managing risks can cause problems. This includes issues with data quality, bias in algorithms, and how we interpret results. These mistakes can lead to bad decisions.
Bridging the Gap: AI and Human Collaboration in Data Analytics
AI and humans work together in data analytics. They each have unique strengths. AI can process information quickly and handle large amounts of data. However, human intervention is important. People help understand the insights, keep things ethical, and make final choices.
1. The Synergy between AI Capabilities and Human Intuition
The mix of AI abilities and human intuition forms strong teamwork. AI is great at handling large amounts of data. However, human intuition is important for understanding results, spotting details, and making ethical choices.
2. Case Studies: Successful AI and Human Collaborative Projects
Many case studies from different industries show how well AI and humans can work together. In areas like personalized medicine and fraud detection, these projects highlight the great results that come from using both sides' strengths to achieve the best outcomes.
Overcoming the Technical Challenges of AI in Data Analytics
Overcoming technical challenges when using AI for data analytics means fixing data quality issues, making sure the models are accurate, and adapting to new technologies and changing data. Continuous learning and solving problems are very important.
1. Addressing Data Quality and Quantity Issues
Effective management of data and AI solutions can solve issues related to data quality and quantity. Cleaning data and checking it carefully can help improve its accuracy. Using synthetic data can also ensure there is enough data to train strong AI models.
2. Ensuring AI Model Transparency and Interpretability
AI model transparency and interpretability are very important for building trust and following regulations. Using explainable AI methods helps users understand better. It also makes it easier to audit and ensures that AI is used responsibly while meeting rules.
3. Scaling AI Solutions for Large-Scale Data Analytics
Scaling AI solutions for large data analytics needs strong technology systems. It also needs smart algorithms and flexible designs. These tools can manage large amounts of data and deal with their complexity in a business setting.
The Economic Impact of AI-Enhanced Data Analytics
AI-based data analytics greatly affects the economy. It helps businesses grow by improving decision-making. It also saves costs through automation and makes market trend predictions better. This results in more efficiency and a better position against competitors.
1. Boosting Business Efficiency and Innovation
Integrating AI makes things work better and drives business creativity. It does this by automating tasks, showing hidden patterns, and supporting decisions based on data. This leads to more productivity. It also helps businesses stay ahead of the competition in a world focused on data.